Data Science in Clinical Care (2024-2025)
Background
Clinical decision support (CDS) tools are data science innovations that aim to provide timely information to help providers make informed decisions and provide the right treatment to the right patient at the right time. While the FDA has introduced guidance to regulate safety and efficacy on the technical side, ambiguity remains about guidance for use in clinical care. For example, CDS tools that predict patients’ events or outcomes using complex algorithms are often not closely paired with recommendations and thus may not have the intended effect of improving patient care or outcomes.
One clinical area that demonstrates this implementation problem is infection after surgery in patients with peripheral arterial disease (PAD), a circulatory condition that results in reduced blood flow to limbs. Because wound infections occur in approximately 20% of patients with PAD, risk scores could identify the highest risk patients and guide implementation of infection reduction measures. However, clinicians vary in how they interpret predictions and actions.
Identifying highest-risk patients is important, because intervening with all patients would require more time and money than typically available. Developing a shared model of care for patients undergoing revascularization for PAD will provide valuable insights to help refine predictive CDS tools and sustainably implement targeted risk mitigation strategies. More research is needed to examine how to effectively implement predictive risk scores to evaluate outcomes in real clinical care settings.
Project Description
This project team will develop best practices for adoption of CDS tools through participatory systems dynamics modeling, a process to engage individuals in developing a common understanding of interrelated factors that contribute to a specific problem and leverage points. In this case, participatory systems dynamics modeling would provide an important tool set for stakeholders to understand how algorithms can be implemented into clinical workflows.
Building on the work of the 2023-2024 team, this team will identify barriers to risk model adoption and bundle implementation to reduce surgical site infections in lower extremity revascularization; generalize a system dynamics model of CDS implementation developed from prior work; and expand training of participatory system dynamics modeling methods at Duke.
Team members will collect data from around 20-30 staff at Duke Health who work with CDS and the use case of lower extremity revascularization. These staff will include CDS developers and owners and clinical providers (e.g., nurses, medical assistants, doctors). Activities will consist of group workshops with staff to iteratively develop models of system dynamics that influence use and effectiveness of a CDS tool to direct intervention after lower extremity revascularization. These workshops will follow participatory system dynamics modeling techniques, also called group model building, to engage participants in developing an explicit, shared understanding of dynamic factors that affect behavior.
In the series of workshops, the team will elicit key variables and their behavior over time from participants, culminating in the development of a causal loop diagram that depicts pathways between variables (direct paths and feedback loops). This causal loop diagram will depict a negotiated, shared understanding by staff of the problem of a disconnect between risk score and targeted intervention.
These findings will be incorporated into prior work to continue to build a generalizable understanding of the problem of CDS adoption. The resulting model will provide a framework for decision makers to understand key factors and leverage points to improve adoption of clinical decision support in a complex system.
Anticipated Outputs
Operational guidance to use risk models for infection risk assessment; best practices for clinical teams; case study of system dynamics affecting risk scores; publications and presentations; computerized model of the problem of CDS implementation
Student Opportunities
Ideally, this project team will include 1 graduate student and 6-9 undergraduate students with interests or backgrounds in data science, engineering, computer science, statistics, business, medicine, sociology and/or public policy.
Students will learn to execute participatory system dynamics modeling techniques (including variable elicitation, graphs over time and causal loop diagramming scripts) through in-person workshops with clinical staff; contribute to ongoing development of a generalizable online simulation model of factors that affect implementation of clinical decision support tools by incorporating findings from this project's use case; and prepare report, papers and presentations to demonstrate the innovative approach to study clinical decision support implementation and communicate findings to operational, academic and policy audiences.
Team members will meet weekly to develop project goals and deliverables and learn new research skills in participatory system dynamics modeling.
Timing
Fall 2024 – Spring 2025
- Fall 2024: Submit Institutional Review Board (IRB) for approval; conduct literature review; conduct training and workshop planning
- Spring 2025: Continue workshops; conduct data analysis; develop simulation model; write reports and papers for publication
Crediting
Academic credit available for fall and spring semesters
See earlier related team, Data Science in Clinical Care (2023-2024).
Image: Sara Schick and Leah Kranja assist a patient in infusion at Duke Women’s Cancer Care Raleigh (Patient is staff posing, Ivey Aronowitz), by Erin Hull/Duke Health
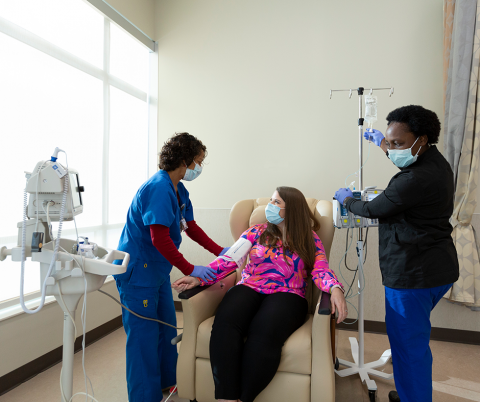
Team Leaders
- Adam Johnson, School of Medicine-Surgery
- Nina Sperber, School of Medicine-Population Health Sciences
/yfaculty/staff Team Members
-
Benjamin Goldstein, School of Medicine-Biostatistics and Bioinformatics
-
Scott Rockart, Fuqua School of Business