
Project Team
Big Data for Reproductive Health (2019-2020)
Team profile by Kelly Hunter, Stephanie Skove, Dennis Harrsch Jr., Anna Hirsch, Janel Ramkalawan, Saumya Sao, Elizabeth Shulman, Eugene Wang, Zhixue Wang and Qintian Zhang
Since its inception in 2018, our team, Big Data for Reproductive Health (bd4rh), led by Megan Huchko, MD, MPH, Associate Professor of Global Health and Obstetrics, Amy Finnegan, PhD, MPA, Senior Data Scientist at IntraHealth International, and Sanford School of Public Policy and Political Science PhD student Kelly Hunter, MA, have endeavored to make data on global contraceptive use accessible to those who need it most: family planning decision makers. Over the course of two Data+ and two Bass Connections projects, we have developed a suite of online visualization tools to do just that, contributing to a booming field of research with similar goals. We hope that our tools can aid stakeholders in directing tailored family planning resources towards key populations, thus helping to improve global sexual and reproductive health.

During the 2019-2020 academic year, the particular focus of our project was to examine the feasibility of implementing a precision medicine family planning counseling tool to support healthcare workers in low income settings around the world. Such a tool would provide customized recommendations for family planning methods based on a person’s demographic and geographic characteristics to the healthcare worker, who would then use those recommendations to counsel the person.
To answer this question of feasibility, our team branched out into several different lines of inquiry. Our stakeholder engagement sub-team, composed of Saumya Sao (Gender, Sexuality, and Feminist Studies & Global Health), Elizabeth Shulman (Computational Health), Janel Ramkalawan (English), and Elizabeth Loschiavo (Visual and Media Studies), assessed the digital family planning landscape and interviewed relevant stakeholders to determine how our team could proceed ethically in this research space. Meanwhile, our machine learning sub-team, composed of Zhixue (Mary) Wang (Computer Science), Dennis Harrsch, Jr. (Computer Science & Global Health), Annie Hirsch (Computer Science), Qintian Zhang (Statistical Science), and Eugene Wang (Computer Science) reviewed and tested big data algorithms for our predictive tool to minimize data quality issues and maximize data accessibility.
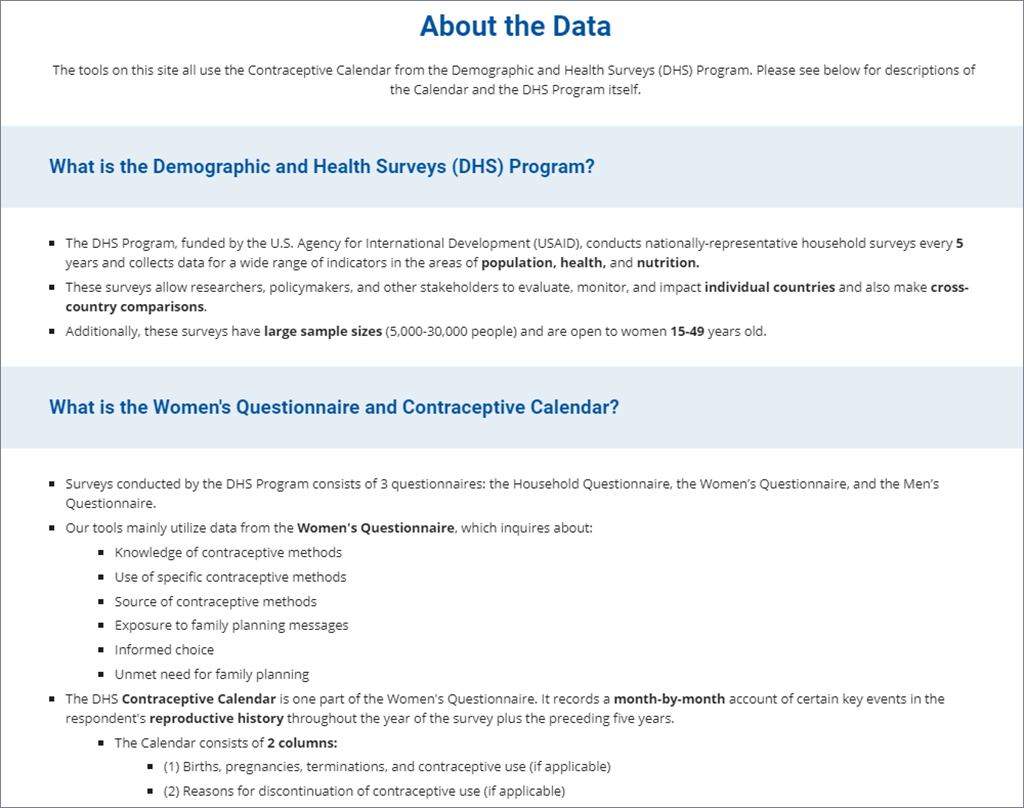
Over the course of these various investigations, our team encountered numerous opportunities to learn about the intricacies of tool development in the digital family planning landscape. First, we discovered from stakeholder feedback our tool suite’s niche as educational apps predominantly suited for decision makers who want to a) understand what contraceptive data is and b) what contraceptive discontinuation and switching look like in a dynamic format. Additionally, we learned the costs and benefits of different big data algorithms, including neural networks, decision trees, support vector machines, and K-nearest neighbors, in modeling such data.
While results showed potential for these machine learning models to accurately fit our complex dataset, we could not discount the value of interpretability found in simpler logistic regression models. From these findings, we began to understand that the feasibility of a predictive tool would depend on intentional, user-focused design. Many existing digital health interventions in this research space were not developed in accordance with best practices for designing sustainable, community-driven technologies. In order for our tool to fulfill its intended purpose, we needed to reexamine the importance of the user in the design process and engage stakeholders in development.
Next year, the Big Data for Reproductive Health (bd4rh) project will enter its third year. Though its focus will be shifting away from developing our tools toward examining contraceptive use dynamics through social media, we hope that the findings and deliverables we have produced this year will benefit not only the broader field of global sexual and reproductive health but also future years of our project. We would like to conclude with a huge thank you to the Bass Connections program for the opportunity that it has afforded us to learn and research.
Please check out our website to view our suite of data visualization tools.
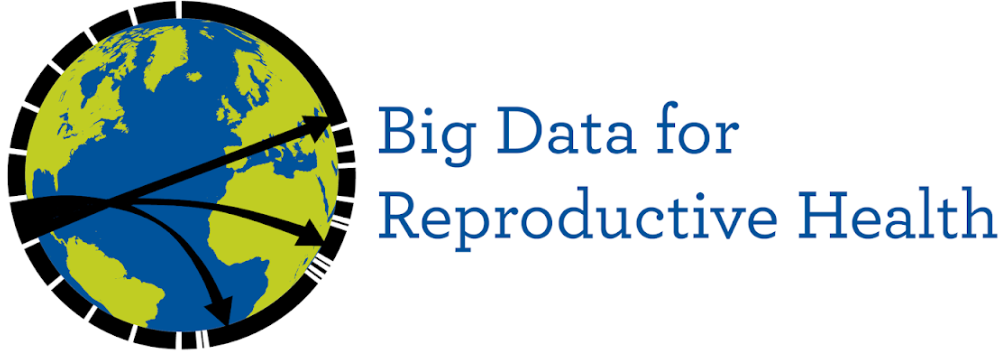